Beyond the essentials: Unlocking value from data
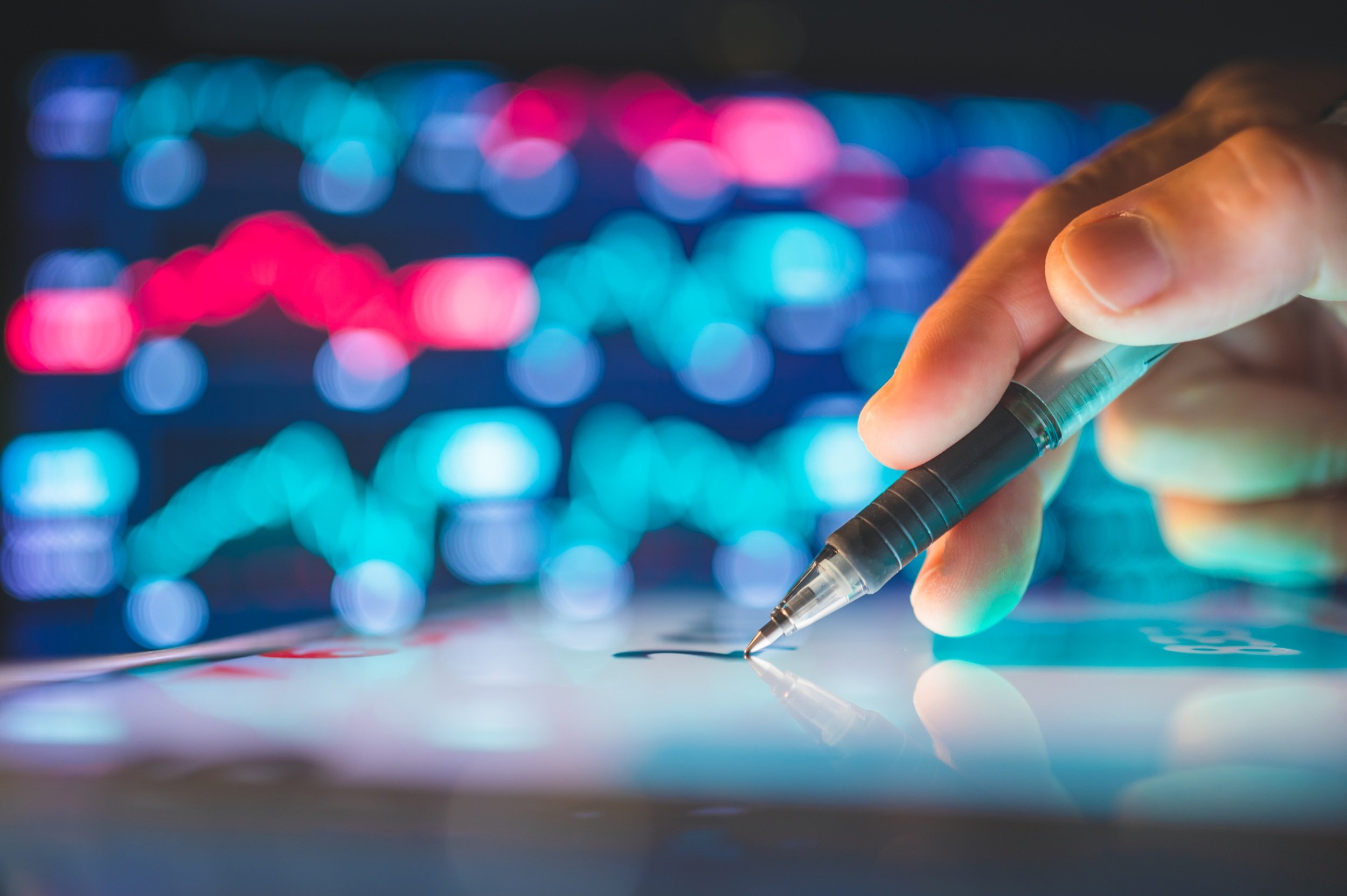
Although data has become one of the most valuable assets for businesses today, many companies still struggle to extract meaningful insights from it. While basic data collection and reporting are now standard practice, the next step is leveraging data for strategic decision-making.
This article explores data applications beyond essential data collection and reporting, including tools like dashboards, data visualisation, and entry-level AI technologies. With these tools, businesses can drive operational improvements, enhance client services, and create a competitive advantage.
The power of dashboards and data visualisation
In today’s digital world, where data is abundant yet often under-utilised, businesses need innovative solutions to transform complex information into actionable insights. Tools like dashboards and data visualisation platforms can help companies quickly identify trends and detect anomalies and opportunities.
Data visualisation is the graphical representation of information using charts, graphs, and maps. As a customisable platform displaying various visual data in one place, dashboards enable businesses to monitor key performance indicators (KPIs) in real time, reducing reliance on static reports and manual analysis.
For example, an accounting firm can use a Power BI dashboard to track audit timelines, ensuring teams complete reviews efficiently. Instead of relying on static reports, companies can get interactive visualisations that identify data patterns and highlight trends, underperforming areas, and revenue dynamics. Armed with these tools that transform raw data into actionable insights, teams across an organisation are better positioned to make operational improvements.
However, AI-driven analytics has the ability to provide businesses with a forward-looking perspective. With this technology, companies can move beyond analysing past data and leverage data to predict future trends and make proactive decisions.
Optical character recognition (OCR) for efficiency gains
Optical Character Recognition (OCR), also called text recognition, is a technology that transforms paper-based or image-based data into digital format so they can be processed and analysed. This is particularly valuable for businesses where large volumes of invoices, contracts, or handwritten notes are part of the workflow. OCR technology can help these companies automate data extraction, reduce human error, and enhance compliance efforts.
For example, retailers can use OCR to scan invoices and receipts, automatically updating inventory and pricing without manual data entry. Logistics companies can use it to extract shipment details from customs documents, accelerate border clearance and minimise paperwork bottlenecks. Finance can automate invoice processing by extracting data from checks and digitising financial statements, reducing manual work prone to human error. In healthcare, OCR can help convert patient records, prescriptions, and handwritten notes into structured electronic health records (EHRs).
Entry-level AI tools for smarter decision-making
When powered by AI, data analytics gives businesses a forward-looking edge, allowing them to move beyond past analysis to predict trends and make proactive decisions.
Today, AI technology is no longer reserved for tech enthusiasts and specialists. Even companies with limited data expertise can implement entry-level AI tools to enhance operations. For example, powerful machine learning algorithms can detect anomalies, predict customer behaviour, and automate routine tasks.
Machine learning is a subfield of AI that enables computers to learn without being programmed. These algorithms identify patterns within datasets, allowing computers to make predictions, automate tasks, and improve over time without human intervention.
Natural language processing (NLP) is one of the most widely applied fields of machine learning. In NLP, computers are trained to understand natural language as spoken and written by humans.
A machine learning algorithm analyses historical data to build a predictive model, generating forecasts when new data is introduced. The accuracy of these predictions improves with the volume of data available, but the quality of data is also essential.
These algorithms are not a recent development—they have been around since the 1950s. What is new, however, is the availability of a vast amount of data—also called Big Data—created daily in our digital world. This massive amount of data fuels complex machine learning algorithms, allowing them to learn and improve their delivered results at a speed and scale never seen before.
Machine learning has been changing or will change every industry in the near future. For example, a law firm can use natural language processing to analyse past case outcomes and predict the likelihood of success for client cases. Businesses can also use it to monitor social media and user sentiments, as well as customer responses and reviews. Machine learning is also the technology that drives recommendation engines and chatbots. In healthcare, these algorithms can be trained to analyse medical data, including images, to detect specific markers of illness.
From fraud detection in banking to personalised recommendations in e-commerce, Netflix and YouTube, machine learning helps businesses transform data into insights and translate insights into action.
As companies rely more on data-driven decisions, understanding the strengths and limitations of machine learning algorithms becomes essential.
While these tools offer immense potential, they are not infallible—they depend upon the quality of the data they are trained on and require careful oversight to prevent biases or errors.
Also, the human element in data analysis is still essential, especially when interpreting results delivered by the algorithms. For example, a well-documented case showed a machine learning algorithm seemingly outperforming doctors in diagnosing tuberculosis from X-rays. However, instead of analysing the images, the algorithm relied on an unintended pattern—associating older X-ray machines (more common in developing countries with higher tuberculosis rates) with positive cases—leading to misleading results.
Case study: Using data analytics to detect fraud in healthcare billing
Let's consider how machine learning can improve the operations of a healthcare organisation.
HLB Mann Judd Australia (HLBMJ) was engaged by a regional hospital to investigate potential irregularities in a medical practitioner's practice. The hospital collaborated with HLBMJ's data analytics team to investigate these concerns using a data-driven approach.
The hospital needed sound evidence to support its suspicions about the irregular practice while maintaining confidentiality. The investigation involved collecting data from various sources, such as operating theatre records and patient billing systems, requiring coordination among stakeholders with different perspectives and priorities. Successfully managing these relationships while preserving the integrity of the investigation was crucial to its success.
Using Alteryx, HLBMJ extracted and cleaned data from various hospital systems, ensuring consistency and accuracy. DAX equations were used to compare recorded theatre time with billed time, flagging discrepancies. Power BI dashboards enabled hospital administrators to see patterns and anomalies in billing practices. Daily check-ins with the hospital ensured the investigation aligned with their needs and allowed for real-time adjustments.
The data analysis revealed that the medical practitioner had overcharged by approximately 1,000 units over 12-month period, amounting to over-billing of around AUD 36,000. As a result, the hospital recovered overpayments and terminated the contract with the practitioner. The hospital also implemented new data validation checks to prevent future discrepancies.
Unlocking your data potential
Tools like dashboards, OCR, and AI-driven analytics can be game-changers for firms at the intermediate stage of data adoption. By implementing these solutions, businesses can achieve higher efficiency in routine tasks and improved decision-making based on real-time insights.
Our Data Analytics & Business Intelligence practice specialises in helping companies unlock the full potential of their data. Whether you’re looking to optimise internal processes or create new service lines, our team can provide expert guidance tailored to your needs. Contact us today to explore how data analytics can drive success for your organisation.
Related content
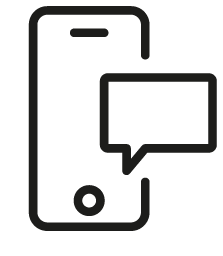
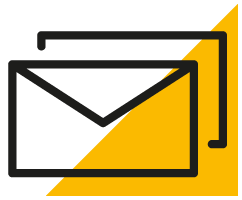